Analytics Must Support Employee Decisions, Not the Other Way Around
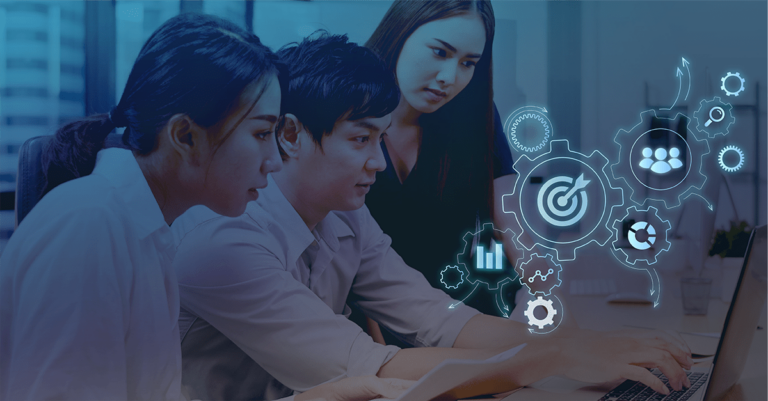
Today’s analytics are so powerful and accessible that they can drive value-based decision-making at all levels of the organization. No matter your industry, applications for analytics are available for any department—marketing, HR, operations, and others—enabling every team member to access and leverage insights they understand.
Most senior leaders believe achieving these results means transformative changes, not only to enterprise technologies but also to the fundamental ways their employees do their jobs. This simply is not the case. Real-world evidence supports the often-counterintuitive idea that new analytics technologies must adapt to employees’ existing (good) habits when it comes to decision-making, rather than employees changing their habits to accommodate unfamiliar tools.
This approach does not reduce the complexity of both analytics implementation and user adoption. After all, “advanced analytics can drive value only if employees use them to make decisions,” as McKinsey describes: “[But] The more familiar and intuitive a model is, the more likely it is to gain acceptance … integrating the analytics into core processes can make new systems feel like a natural extension of existing ones, rather than an abrupt change.”
Here we take a closer look at how analytics can align with existing, effective workflows, thereby helping employees in their decision-making roles rather than forcing them to change. We will explore the key elements that make up a successful modern analytics environment as well.
Modern Analytics Must “Fit In” With Existing Best Practices
A recent Korn Ferry report identifies one of the greatest workforce challenges of 2021: “needed capabilities are changing faster than it’s possible for organizations to shift their workforces.” That’s why leading companies are attempting to bring facts-based decisions into all levels of the organization. They are “democratizing” access to advanced analytics as part of a “business decision supply chain.”
The business decision supply chain can be defined generally as a sequence of processes by which raw data is transformed into trusted, actionable insights that everyday business users can leverage for each of their own unique purposes. In the right environment, these insights not only provide the best value for each employee’s decision-making but are also easy for that employee to access and understand—no heavy technical training required. The result is twofold:
- Employees are happier—they are better at their jobs with less guessing and manual work.
- Results per employee are better across the enterprise, driving business value and resiliency.
Making employees happy seems like a small goal for enterprise technology, but it’s actually critical to securing consistent use of analytics tools throughout the organization. The most powerful analytics are useless if users don’t feel the need or desire to use them.
The Three Key Elements to Successful Analytics
Good employees don’t live in a vacuum, either. They are aware of the business challenges surrounding their organizations and are most often open to some degree of change. With that in mind, we’ve identified three key elements that contribute to a successful, data-driven decision-making environment: in the subject areas of people, processes, and technology. If you’re early in analytics adoption, consider how to approach these three factors as you begin.
1. Help People Decide and Adapt to Market Changes
In practice, individual teams and the initiatives with which they are most familiar shape analytics success for organizations. Leading companies are working to instill a profound sense of trust in analytics capabilities among their employees as a result.
But business leaders must proactively understand the needs of those specific teams before deciding which data resources are right for them. They should maintain the essential goal of helping those employees in their decision-making—rather than forcing change alongside new technology adoption—as they move forward.
Korn Ferry highlights an example of this change in their report, namely the changing role of a hypothetical salesperson. With fewer in-person visits to clients, her focus has shifted to become more data-focused: Today, she builds compelling proposals for clients using analytics. But while the salesperson’s focus has shifted, her responsibilities and KPIs have not fundamentally changed. She simply has more resources at her disposal, even if the broader business climate has reduced her opportunities to engage clients face-to-face.
2. When It Comes to Processes, Integrate and Improve
In practice, integrating analytics is about keeping “what works” for employees—processes they prefer and that drive results. For example, marketers can transition away from subjective decision-making and begin thinking strategically based on a wide variety of data-driven insights. Their responsibilities have not changed, but the ways in which they drive value are more accurate, and they are more confident.
McKinsey cites another example—that of a mining company where dispatchers and operators were subjected to an alarm they most often ignored. That’s because the alarm did not fit into their existing workflow and therefore served no practical purpose. It was by “embedding the alert mechanism directly into the [particular] monitoring system” that those workers found it useful as the alert coordinated with their preferred processes.
3. Make Sure Your Analytics Technology Has “Legs to Stand On”
As you consider analytics platforms, remember this: All the best capabilities in the world won’t matter if employees don’t use the tools. How employees access and use analytics are therefore foundational to adoption and ultimately, ROI. Analytics environments that lend themselves to this level of adoption are critical as a result.
Realize Business Value, and Employee Success
New technologies always involve learning requirements. But the fundamental advantages of existing processes and goals that employees have come to value must be enhanced, not eliminated. Data leaders must consider both user and business needs and then assign data resources and self-service capabilities to employees with those in mind. That’s how the business decision supply chain becomes truly successful, transforming data into real business value.
This post originally appeared on PyramidAnalytics.com